In a world inhabited by a multiplicity of truths, how may we develop mechanisms to orient positively therein?
This article explores knowledge graphs as a tool for sensemaking and epistemic pluralism. Data is not neutral. It is contextual — collected under particular conditions, by particular people. And when we gather data together and attempt to make sense of it, we add meaning to it to produce an insight. Insights can then be repackaged as new data points within a layered graph. Rather than seeing these insights and data points as neutral fact, we explore the way that knowledge is a diverse field, with overlapping interpretations. By building tools that let us see these divergences and track their origins, we believe that we can better enable community and society level sensemaking.
Making Sense of Contextual Data
Raw data points alone are meaningless without interpretation. They exist as inert quantifications exerting only the direct geometries of their measurements and have no further relation to reality. The quantity of lead particulates in public water systems alone is insufficient to derive meaningful action. First, we must also understand that lead particulates are dangerous to humans when consumed and that humans are dependent on public water systems to survive.
Insights — defined for this post as artefacts of knowledge — are always in relationship to an ontology; they exist within a framework for sensemaking reality. Thus data points and their descendant insights can be divergent across ontological frameworks. The challenge then — for us as a collective species interdependent with the natural world — is to develop a system within which we can make decisions that benefit us as a whole and enable us to cohere towards some common goal, despite our differing opinions, interpretations, agendas, and means of engaging reality. What is more, we must be able to transcend a mono-ontology to truly understand the complexities in the hearts and minds of the entities that inhabit Earth.
It is the composition of measurements into published, opinionated claims that thread the fabric of a ‘knowledge topology’ upon a distributed library of available data.
Gesturing Towards Pluralistic Knowledge
Recognizing the pivotal role of cogent insights in informed decision-making, it becomes imperative to establish a system that can both acknowledge and effectively evaluate a diverse array of perspectives, especially when they range from congruent to starkly divergent. This necessity stems from an understanding that our collective perception of the world is inherently pluralistic, encompassing a spectrum of contexts and ontological frameworks where multiple rich contexts and ontologies need to be able to coexist and organise towards a future that is inclusive, yet coherent, interdependent, self-aware, and common enough such that we can avoid undesirable internecine interactions.
It is here that a set of tooling in support of a healthy, composable and pluralistic Data Commons is described, or more accurately, a set of tooling to enable data commoning! This tooling extends the distributed data storage, query, and analysis modules described in part one of this blog series.
Adapting our collective lens for knowledge
The authors do not intend to describe a specific implementation. Rather, this post serves as an investigation into praxes of pluralism and knowledge integration. The exact form of these praxes codified into a product are not the focus of this enquiry, and rather we wish to draw attention to the dynamics this tool may embody.
While modern science boasts remarkable capabilities in accurately measuring various aspects of our world, this informational chapter of the Anthropocene has repeatedly shown that absolute truth is elusive. This observation does not undermine the pursuit of objectivity in our understanding of reality. Instead, it highlights that the perspectives that shape our collective cultural narrative are diverse and often lead to substantial variations in our conceptual models of reality. This diversity underscores the importance of acknowledging and designing an effective lens for the plurality of views when engaging in our collective quest for knowledge and understanding.
Consider the following diagrams, where two polar (and very generic) ontologies are described. The authors do not intend to provide an argument for either ontology, but rather give them by way of example. The first is a theistic epistemology, the second is an atheistic epistemology. The nodes in both diagrams are shared data points. The highlighted edges represent the structural relationships between the nodes for each epistemology. The semi-opaque edges represent an opposing ontology and have been rendered for the purpose of demonstrating the pluralism that is the thesis of this post.
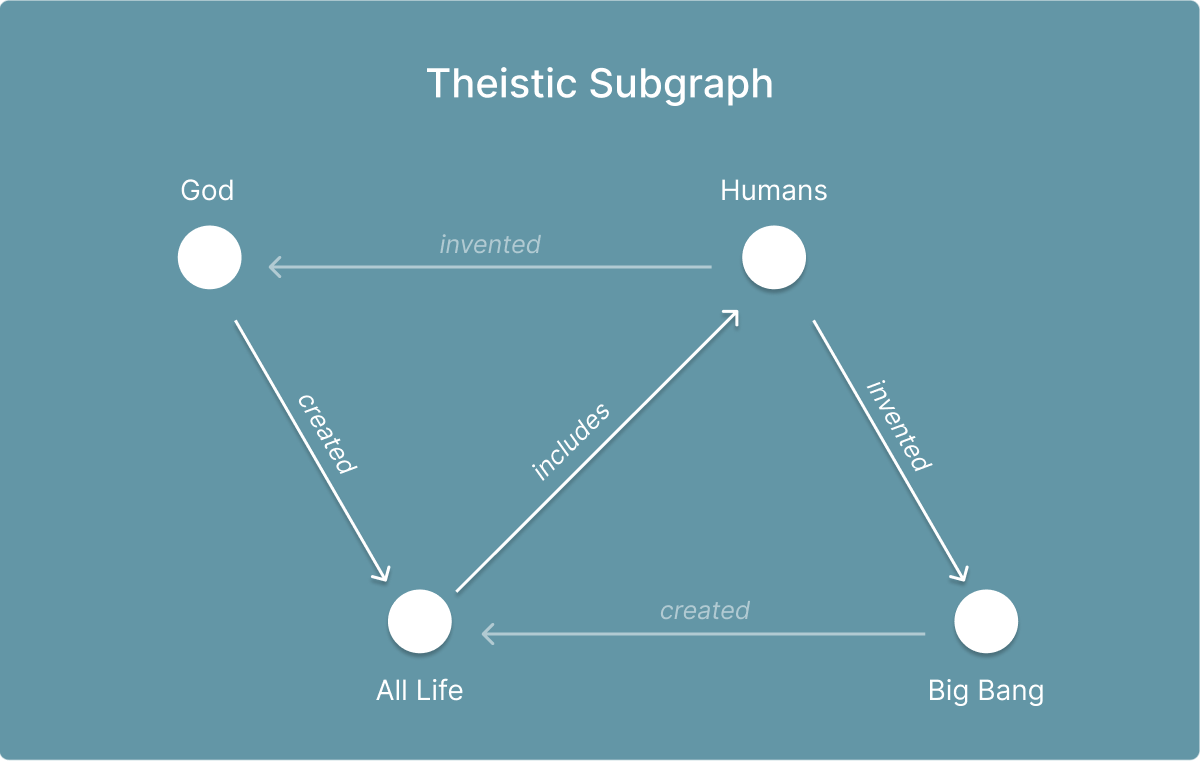
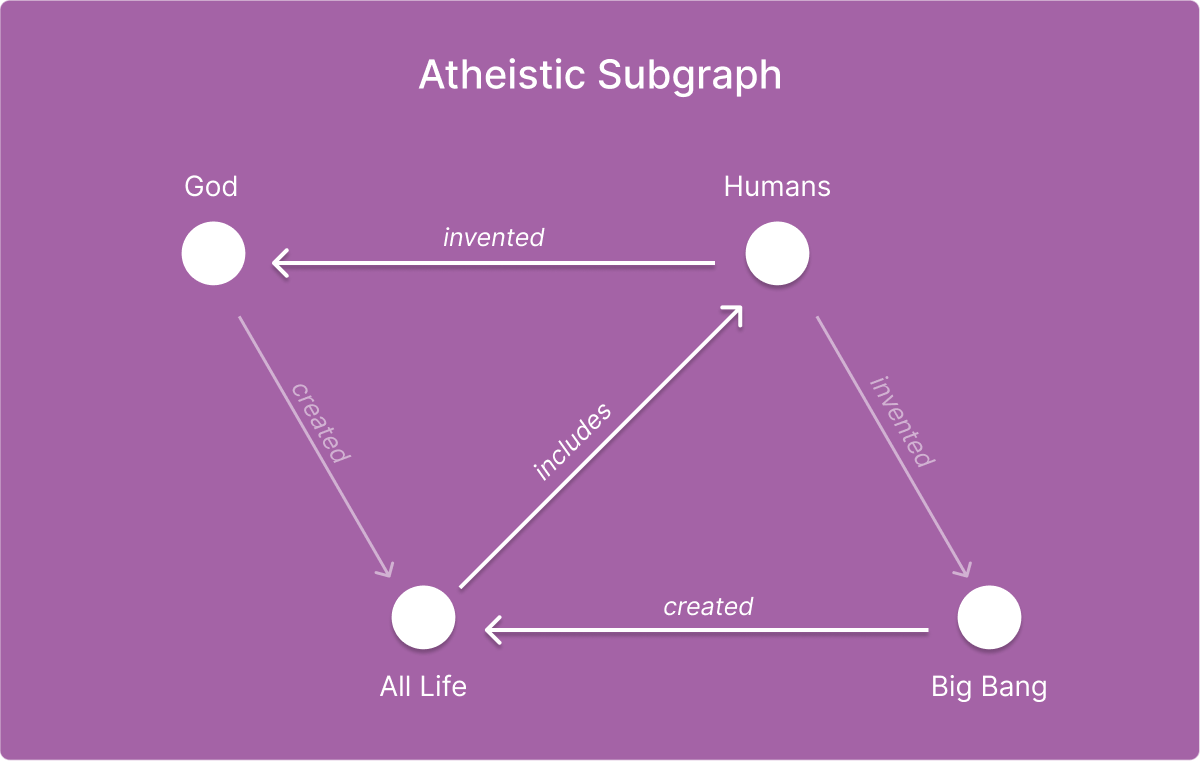
In the above two diagrams, four configurable ‘insights’ are represented by very simplified nouns. Further, the use of simplified concepts such as God, Humans, Big Bang and All Life are intended to represent more complex composable insights and have been selected for their capacity to demonstrate how the epistemological topology of a community can vary despite using the same nodes. Specifically, it is the relationships between the nodes — their relative structure — that determines their framing and ultimately their meaning within a system of knowledge.
The purpose of the above illustrations is to demonstrate that even with some shared understanding of a set of data points (where both ontologies acknowledge the existence of “humans”), it is the relationship of these data points to other data points that define their ontological framing.
Epistemic Pluralism and Peer-Based Graph Structures
Software can act as a bridge connecting us to — and mediating our interactions with — the physical world. It can also oversimplify and potentially degrade our experience of reality. This dichotomy underlines the importance of our mission: to design technological solutions that enrich, rather than diminish, our engagement with the world. Our aim is to ensure that these technological interventions augment our understanding of reality, maintaining its depth and complexity in a way that unlocks the rich and existing knowledge mappings that percolate in our brains, epigenetics, culture, and shared interpersonal space.
With the above framing in mind, we can begin to gesture towards a pluralistic knowledge graph of insights; a multiplicity of complex claims with directed links (references) to the datasets upon which the claims are based.
Enabling pluralism within a tool for knowledge is non-trivial. The challenge is to determine and promote synthesis and coherence across an agent-centric knowledge mapping tool.
The reader is encouraged to imagine a graph-builder of sorts, where participants provide insights via some medium as nodes in a graph. These publications will bear some epistemic relationship to other publications. They may be direct responses, endorsements, or rebuttals but they may also have less explicit relationships such as semantic similarity or geopolitical proximity. It is this metadata, the edges of the graph or in other words its relational structure, that represent the social relations through which we have collectively archived and evolved our various cultures for millennia.
Designing for Protocols > Platforms
This blog post does not delve into the specific design or user experience (UX) of the proposed tool. Instead, our focus is to conceptualize its potential functionalities, particularly when implemented as a protocol for safely sharing data and insights across software ecosystems. We envision it as a flexible module or wrapper, designed for integration by other developers, rather than as a standalone platform. This approach considers an inter-platform collaborative model. A key aspect of this model is the 'safe answers' concept as examined in part one of this blog series, which negates the need for centralized server access to raw data. Instead, it enables communities to retain custodianship over their data, allowing external organizations to send localized algorithms to peer networks. These algorithms can then query data, with granular control over the opacity of the data being used for computation. This means that the return of the algorithm can optionally expose the raw data it is using, or provide some ‘insight’ pertaining to the data. For example, a security camera may expose to a querying party the amount of people who walked past the camera, and not the identity/profile of the individuals walking past. This method fosters a decentralized and secure approach to data analysis and sharing.
Finding Integration in Pluralism
While there is something just and encouraging about an ecosystem aimed at mapping the pluralistic epistemics that percolate throughout the world, there are also risks therein. What is important is that we avoid a set of disintegrated ‘knowledge’ feedback loops, echo chambers, where those embroiled in misguided or harmful informational clusters are never exposed to counter-arguments or differing schools of thought. It is important that we identify mechanisms through which to measure how coherently any given network’s graph of knowledge integrates with the other graphs in the ecosystem. To achieve this, we endeavour to draw from Integrated Information Theory (IIT).
IIT, initially formulated to describe consciousness, posits that a system possesses consciousness to the degree it possesses 'Phi' (Φ) — a measure of its intrinsic cause-effect power. It is a system's ability to integrate and differentiate information. Adapting this to our knowledge graph setting, we can interpret 'Phi' as a measure of the extent to which knowledge from one epistemological network is both differentiated from and integrated with another. The higher the Φ, the more integrated (and thus potentially coherent) the knowledge graph is with the broader knowledge ecosystem.
In practice, Φ along with more traditional graph analysis techniques may be used to reveal areas where connectivity can be enhanced, subsequently tuning search and discovery algorithms. This is crucial in a world increasingly fragmented by differing worldviews, as it enables us to identify points of convergence and divergence across knowledge systems. By analogizing Φ in IIT to the integration of knowledge across different epistemic frameworks, we establish a quantitative measure and orienting function to shift the knowledge network toward greater coherence.
Just as IIT posits that conscious experience arises from the integration of differentiated information within the brain, we posit that a coherent and functional knowledge ecosystem could emerge from the integration of differentiated insights across various epistemological networks. We suggest that a healthy knowledge graph, like a biological mind, is not just a repository of information, but a dynamic, integrated network capable of evolving and adapting to its environment.
Embracing the Dynamics of Knowledge Integration
The journey through the realms of data, insights, and knowledge graphs has elucidated the intricate and dynamic nature of knowledge itself. We believe that the concept of a pluralistic data commons, as explored in this article, is not just a theoretical framework but a necessary evolution in our approach to understanding and navigating the complex tapestry of global information. The notion of knowledge as a static, singular entity is a relic of the past. Today, we are called upon to recognize the fluidity and multiplicity of knowledge, shaped by diverse perspectives and evolving contexts.
This blog post describes praxes for knowledge mapping in a manner that aims to embrace epistemic pluralism, and resists the idea that we as a species can arrive at a positive orientation through a homogenised narrative. Rather, we believe that it is in the intersection of a diversity of narratives that we may come to a model of integrated knowledge. These praxes are important when considering the applications that may sit above the distributed storage layer we described in our first blog post. It is insufficient to merely ‘capture’ data. Data requires contextualisation and application, and it is in these later steps that the capacities and implications of the data becomes apparent. Here we must ensure that our endeavours to synthesise and unpack data are done in an inclusive and life-centric manner, and thus it is important that the communities using the distributed storage tool have the means to find the degree of integration in the knowledge artefacts derived from a given set of data.
The notion of measuring the integration of knowledge, borrowing from Integrated Information Theory, offers a promising path forward. It allows us to quantify and visualize the interplay of different knowledge systems, identifying points of convergence and divergence. This, in turn, can guide efforts to enhance connectivity and coherence within the global knowledge ecosystem. Such an approach recognizes that the health of our collective understanding is not just in the accumulation of data or insights, but in the integration and harmonious interplay of diverse epistemologies.
Ultimately, the success of this endeavor will depend on our collective willingness to engage with and evolve differing viewpoints, to challenge our own assumptions, and to remain open to the evolving nature of truth. The creation of these knowledge ecosystems is not just a technical endeavour but a cultural and intellectual one, requiring ongoing dialogue, collaboration, and a shared commitment to exploring the multifaceted nature of reality. As we forge ahead, let us embrace this complexity, not as a hindrance, but as a rich tapestry of human experience and wisdom, from which we can all learn and grow.